RESEARCH
Current Areas of Study
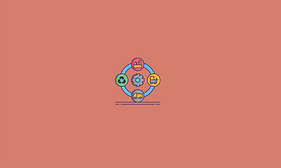
Complex Supply Chains
We work on the development of supply chain algorithms with view to integrate manufacturing uncertainties in planning & scheduling decisions.
We are particularly interested in rapidly evolving supply chains, where facilities are challenged by in-risk manufacturing. In this case, we identify risks related to material & route availability, demand uncertainty and multiscale dependencies.
Our research has its fundamentals in Mixed Integer Programming and Optimisation, augmenting to Machine Learning as means to decrease model complexity.
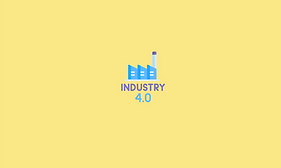
Integrated Process Design and Optimisation
We are pioneering the development of a model-based framework for the simultaneous design, optimisation and control of manufacturing units, considering inter- and intra- process uncertainties. The framework and tools in this space are tackling a fundamental question; "How can we use computer modelling tools to make processes environmentally and economically sustainable by design?". We work in the interface of Engineering, Manufacturing and Digitalisation to enable process digitalisation and integration via continuous monitoring. In this space we are interested in combining design with operational decisions towards the identification of flexible process design that guarantee products with specs.
​
We use mathematical models (mechanistic, hybrid and fully data-driven) as the backbone of the framework and tools under study and we harness the potential of virtual experimentation for a full-scale analysis of the system at hand. The output is a set of design spaces that includes process alternatives that meet the pre-specified constraints.

Model-based Control
A key area of research in our lab is model-based control. The development of advanced, model-based control strategies is a challenging task primarily due to: (a) model complexity and (b) unavailability of online and suitable feedback measurements. These are well-known challenges, often responsible for the slow adaptation of digitalisation and automation in process industries. For this, we work on the integration of suitable digital analogues (digital twins) with the process at hand to provide continuous process monitoring and develop soft sensors to deal with measurement. We focus on the development of advanced, model-based control policies for complex systems (e.g. separation systems). This includes the investigation of different modelling techniques, such as Machine Learning, that can decrease the computational cost of the mechanistic model.
We are particularly interested in control methodologies for:
- End-to-end, plantwide control
- Separation systems
- Improved task scheduling